Supervised learning is a cornerstone of machine learning, providing a structured framework for predicting outcomes based on labeled training data. This method is pivotal for many applications, from financial forecasting to image recognition. In this article, we explore the fundamentals of supervised learning, its types, and the key algorithms that drive its success.
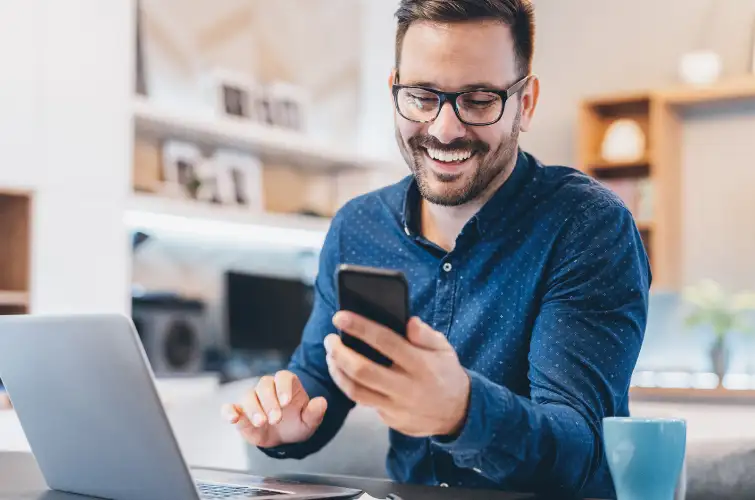
What is Supervised Learning?
-
Supervised learning is a type of machine learning where the model learns from labeled training data to make predictions or decisions without human intervention. The primary goal is to infer a function from the input-output pairs provided in the training set, which can then be used to map new, unseen data to the correct output.
Key Characteristics:
- Task-Focused: Supervised learning is specifically designed to address tasks like predicting numerical values (regression) or categorizing data into classes (classification).
- Function Inference: It aims to determine the mapping function that best fits the input-output relationship from the training data.
- Labeled Data: The training data is labeled, meaning each input is paired with the correct output.
- Data Distribution: Ideally, the features in the data should be independent and identically distributed (i.i.d.). However, in practice, training datasets often have correlated features with varying distributions.
- Data Sufficiency: The quantity and quality of training data significantly impact model performance. According to the Good and Hardin conjecture, the dataset size N=mnN = mnN=mn (where mmm is the number of features and nnn is the number of samples) provides a rule of thumb for sufficient data size.
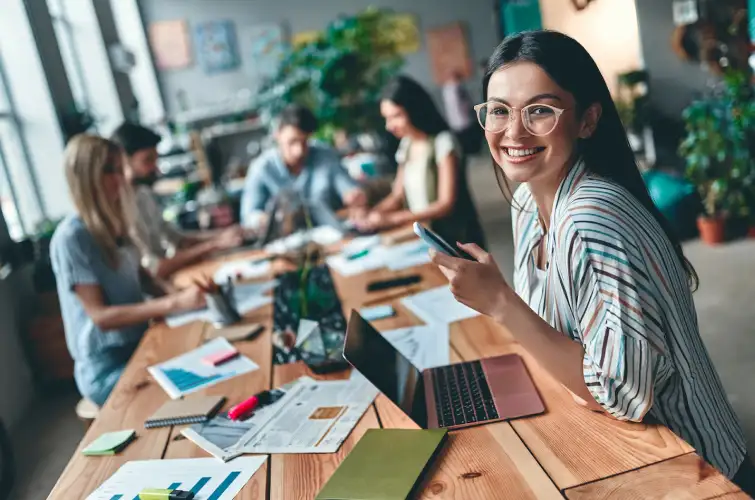
Types of Supervised Learning
Supervised learning can be broadly categorized into two main types: regression and classification.
1. Regression
Regression algorithms are used to predict continuous numerical values based on input data. They are instrumental in scenarios where the output is a real-valued number.
Common Forms of Regression:
- Linear Regression: Fits a straight line to the data to predict the output.
- Polynomial Regression: Fits a polynomial curve to capture more complex relationships.
- Complex Regression: Uses advanced techniques to handle more intricate patterns in the data.
2. Classification
Classification algorithms predict the categorical label or class for input data. These algorithms are essential in applications where the output is a discrete category.
Types of Classification:
- Binary Classification: Distinguishes between two classes, such as spam or not spam.
- Multi-Class Classification: Handles more than two classes, such as classifying images into categories like dogs, cats, and birds.
Types of Supervised Learning
Supervised learning can be broadly categorized into two main types: regression and classification.
Support-Vector Machines (SVM)
Overview: SVMs are used for both classification and regression tasks. They work by finding the optimal hyperplane that separates different classes in the feature space.
Applications: Image classification, text categorization.
Linear Regression
Overview: This algorithm models the relationship between a dependent variable and one or more independent variables using a linear approach.
Applications: Predicting housing prices, stock market trends.
Logistic Regression
Overview: A statistical method for analyzing datasets with one or more independent variables to predict a binary outcome.
Applications: Binary classification problems like email spam detection, disease diagnosis.
Naive Bayes
Overview: Based on Bayes’ theorem, this probabilistic classifier assumes strong independence between features.
Applications: Text classification, sentiment analysis.
Linear Discriminant Analysis (LDA)
Overview: LDA is used for dimensionality reduction and classification, focusing on separating classes by maximizing the distance between the means and minimizing the spread within each class.
Applications: Face recognition, bioinformatics.
Decision Trees
Overview: Decision trees use a tree-like model of decisions and their possible consequences, including chance event outcomes and resource costs.
Applications: Customer segmentation, medical diagnosis.
K-Nearest Neighbor (KNN) Algorithm
Overview: KNN is a non-parametric method used for classification and regression by comparing new input to